with b=1.9 and an initial rate of cooperation
among population of Ci=0.5.
We have here parameters of a chaotic regime in the
original model (cf annexe 1). Contrary
to what happen in the case of a society with only Maxi-agents,
there is no chaotic regime but rather an organisation of the network
with specific structuresfor each rule for imitation.
The following graphs show the evolution of a particular
simulation. Most simulation results are shown until time step 300 since
dynamics don't change any more after this time. The most interesting
phenomena take place at the beginning. The first striking thing is that
the system reaches very quickly its unique attractor (20 periods), which
is mostly static (for a 10000 agent population, about a hundred oscillators
at the level of imitation rules, less than a dozen at the behavioral
level (see fig
1). In the following, we will first adopt a local point of view,
looking at a particular agent oscillating between several rules for
imitation. We will then have a global approach with statistics on the
whole population.
The particular case of AUDOU
As is was mentionned above, there is still few agents
which keep changing their rules for imitation or their actions even
after the system has reached its attractor. To understand this phenomenon,
we will take the particular case of agent AUDOU. AUDOU is a D-agent
which always hesitate between adopting the conformist rule or the
Maxi rule. As it can be seen on figure 2, AUDOU is at the border
between an conformist area and a Maxi area.
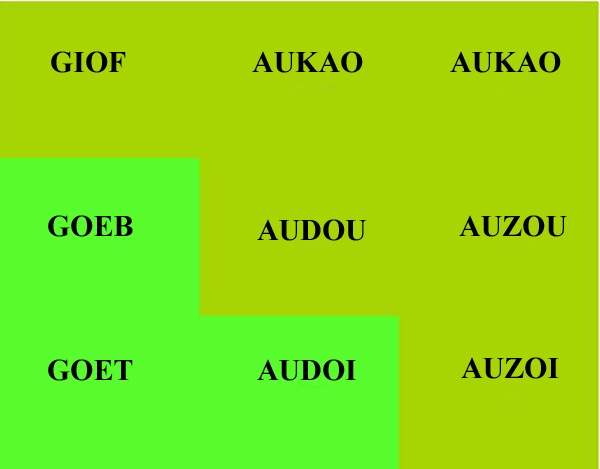 |
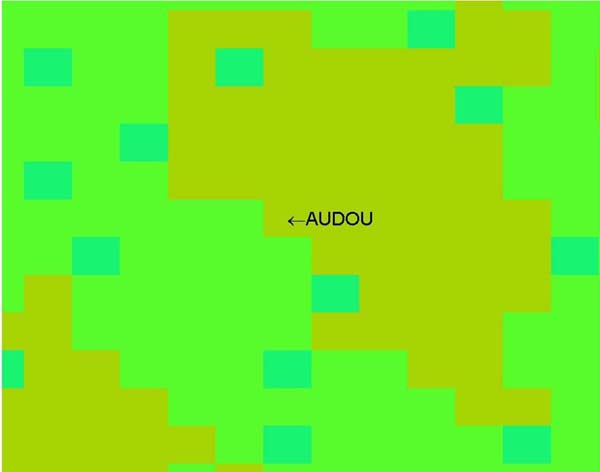 |
Figure 2-a : AUDOU neighbors. |
Figure 2-b : AUDOU, a Maxi-agent is at the border between
an conformist area and a Maxi area. Blue squares are
minority-agents. |
During the time AUDOU keep changing her mind about
how she should choose her action, passing from a Maxi rule
to an conformist rule an so on (fig
2). It is easy from fig2-a to guess why AUDOU can't keep the conformist
rule : in her neighborhood, conformist-agents are always in minority
so that if she adopt the conformist rule, she will change her rule
for the majoritarian rule in the next update. This is confirmed by study
of the evolution of proportions of the different rules in the neighborhood
of AUDOU with time (fig3).
To understand why AUDOU cannot keep the Maxi choice, we need
to look at AUDOU's neighbors payoffs (fig
4 ). What can be seen, is that there are two agents in AUDOU's neighborhood
which are always more successful than AUDOU : AUDOI and AUZOI. Thus,
when AUDOU applies the Maxi rule, she imitates randomly one
of these two agents (since they both have the same payoffs 2). This
explains how AUDOU can choose anconformist rule while beeing adopting
the Maxi rule. We can see here that the topology of the social
network is crucial for this kind of phenomena. It is precisely because
AUDOU wants to imitate some neighbors without having the same neighborhood
and thus, the same information, that she always hesitate between two
rules. The fact that this kind of configurations can only happen at
the border between two areas (of homogenous behaviors or metarules)
with the fact that the whole population is strongly structured explains
why there are so few oscillators.
A Statistical Study of the Dynamics.
The first interesting thing to look at is the frequencies of the different
types of rules for imitation (fig
5 ):
-
The majority rule conformist is actually the
most common in the population at the attractor. This can be understood
by the fact that the higher is the proportion of conformist,
the higher is the probability that an conformist agent will be
surrounded by a majority of conformist agents, which will make
her keep the conformist choice. conformist is self-reinforcing
at the population level.
-
Maxi agents form clusters in the conformist
Sea. Since locally they are actually the ones winning the more,
they don’t change their type.
-
Non Conf. agents are scattered on all the
territory (small dots). Their proportion starting from 25% has decreased
until each Non Conf. agent is actually in minority in her neighborhood.
Non Conf. don’t change their type at the attractor.
-
PDR agents have completely disappeared.
Since for a stochastic rule there are always agents changing their
type for the type of some neighbor, when the other three types have
found stable configurations, the proportion of PDR keep
decreasing until it reaches 0.
Even if this model is a very simple application
of our formalism, it is good news that conformist is actually a very
common rule at the attractorj, since conformism seems to be one of the
most common rule in human behaviours.
If we now look at the spatial distribution of the agent’s modifiable
features (fig. 6), we can notice a very strong structuration with the
formation of clusters of the different types.
We have then that K(i)=1 if the spatial distribution of type
i is random. K(i)>1 means that i-agents
tend to form clusters. K(i)<1 means that i-agents
tend avoid each other. On fig.
7 , we can see the evolution of the rate of clustering for each
imitation rule. The mean clustering rate for imitation rules like Maxi
is 2.8 and 3.6 for cooperative strategies, giving evidence of spatial
structuration of the population.
T he correlations between the imitation rule used by an agent and his
behaviors can be seen in fig
8 . The only cooperators are either anti-conformist
agents (with a mean rate of cooperation of .97) or conformist
agents (with a mean rate of cooperation of .04). Anti-conformist
agents seem to have some role in stabilizing conformist
clusters of cooperators. They occupy positions at the border of these
clusters where C-behaviors are in minority, stabilizing this
border. Without these Non Conf.-agents, these clusters should have
been much smaller.
If we now look at the mean payoffs per imitation law
(fig.
9 ), we see that Maxi-agents are actually the one winning
the most, but not that much with these settings. On the other hand,
cooperative behaviors are winning the most in mean (fig.
10 ). This is due to the fact that a D-agents surrounded
by other D-agent wins nothing (cf. the matrix of the game).
There are thus large clusters of D-agents winning nothing.
To see this, we can see the spatial distribution of payoffs (fig. 9).
We can notice, comparing fig 6 and fig 11, that red areas (highest payoffs)
correspond to clusters of cooperators. In the perspective of emergence
of cooperation, this result is interesting and show how in the meta-mimetism
framework, cooperation can appear locally with the formation of small
clusters which will have an evolutionary advantage. The spatial structure
of neighborhoods and cognitive capacities of agents in selecting theirs
partners and strategies are however too poor in this particular model
to give further conclusions on this topic.
|
|
Fig. 11: Spatial
distribution of payoffs. Blue : lowest payoffs, red : highest
payoffs. |
|
We made the precedent study for the initial rate of cooperators Ci
ranging between 0.1 and 0.9 and for b in
[1.2 , 2.5]. 3D graphs from this study can be found in Annexe
3 .
© David Chavalarias 2003